In the banking industry, managing risk has become increasingly complex. As financial institutions face new challenges, the need for accurate and timely risk assessment has never been more critical. Big Data analytics has emerged as a powerful tool in this domain, offering banks the ability to process vast amounts of information to identify, assess, and mitigate risks more effectively. By leveraging Big Data, banks can enhance their decision-making processes, reduce losses, and ensure regulatory compliance, making it an indispensable asset in modern banking.
Introduction to Big Data in Banking
Big Data is reshaping the banking landscape by providing unprecedented insights into various aspects of risk management. Banks can now analyze large datasets in real time, enabling them to respond swiftly to emerging risks. This shift towards data-driven decision-making is not only enhancing the accuracy of risk assessments but also improving overall operational efficiency in the banking sector.
Why Big Data Matters in Modern Banking
The importance of Big Data in banking cannot be overstated. It allows banks to analyze customer behavior, market trends, and economic indicators, all of which are crucial for making informed decisions.
In today’s competitive environment, banks that harness the power of Big Data can gain a significant advantage by reducing risk and improving profitability. This data-driven approach is becoming essential for managing the complexities of modern banking, particularly in areas such as credit risk, fraud detection, and regulatory compliance.
The Role of Big Data in Risk Assessment
Traditionally, risk assessment in banking relied heavily on historical data and statistical models. However, with the advent of Big Data, banks can now incorporate real-time data from various sources, including transactional data, customer interactions, and even social media. This shift from traditional methods to a more data-centric approach allows banks to build more accurate and dynamic risk models, helping them to identify potential risks sooner and take preventive measures.
Enhancing Credit Risk Assessment with Big Data
Credit risk assessment is one of the most critical functions in banking, and Big Data is playing a pivotal role in transforming this area. By integrating diverse datasets, banks can develop more nuanced risk profiles for borrowers, leading to better decision-making and reduced defaults.
Building More Accurate Risk Models
Big Data enables banks to build more accurate risk models by incorporating a wide range of data types:
- Transactional Data: Provides insights into a customer’s spending habits and financial behavior.
- Behavioral Data: Helps understand a customer’s Creditworthiness based on their online activities and social media presence.
- Market Data: Allows banks to factor in economic trends and market conditions into their risk assessments.
By combining these data sources, banks can create more comprehensive risk models that reflect a customer’s true financial health.
Real-time Credit Scoring
One of the significant advantages of Big Data in credit risk assessment is the ability to perform real-time credit scoring. This process allows banks to:
- Assess Creditworthiness Instantly: By analyzing up-to-date data, banks can make quick decisions on loan applications.
- Identify High-Risk Borrowers: Real-time scoring helps banks spot potential defaulters early, allowing them to take preventive measures.
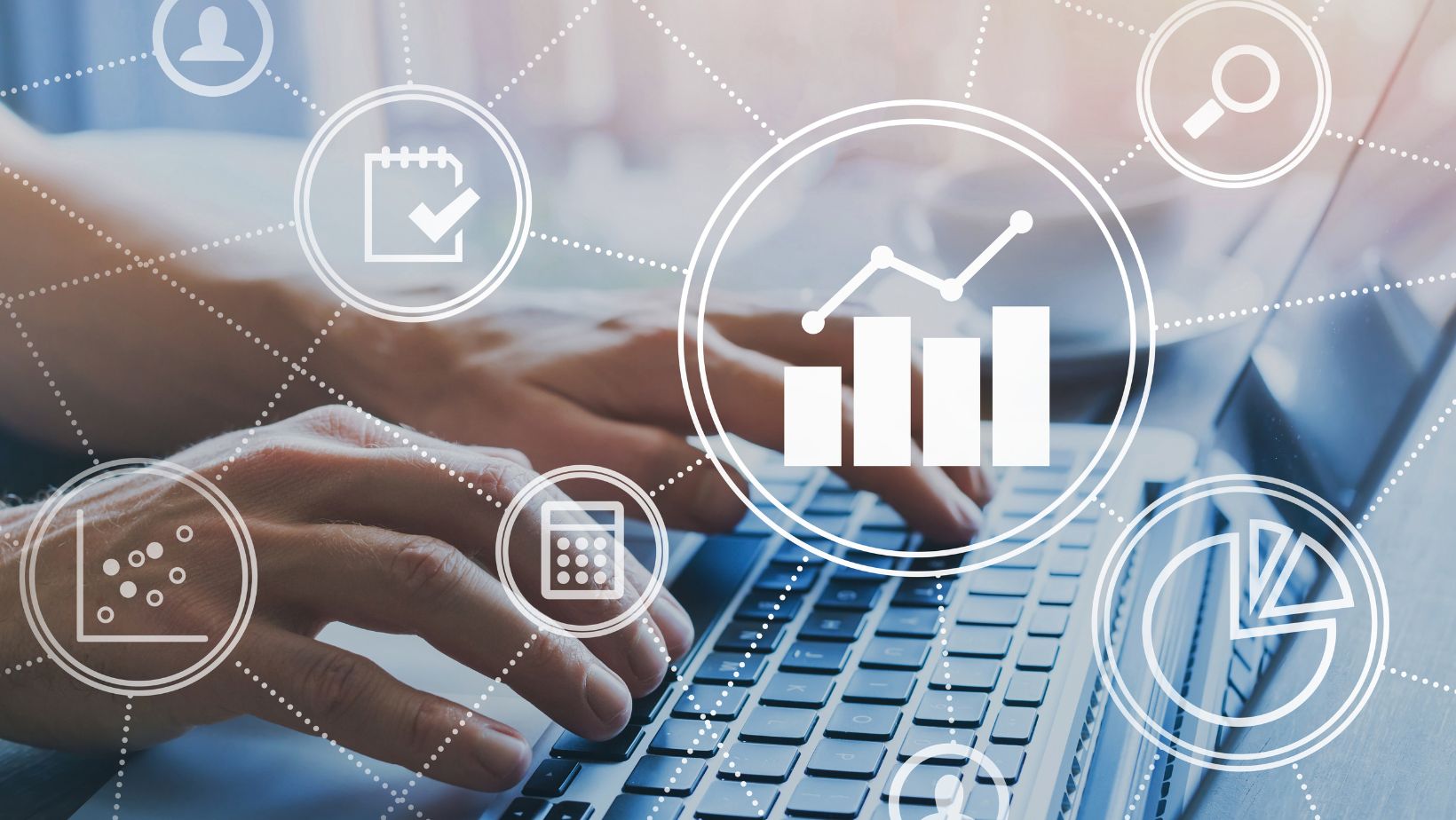
For example, banks can use real-time data to adjust a customer’s credit score based on recent financial activities, ensuring that their risk assessments are always current.
Fraud Detection and Prevention through Big Data
Fraud detection is another area in which Big Data is making a substantial impact. With the increasing sophistication of fraudulent activities, banks need advanced tools to stay ahead. Big Data analytics provides the solution by enabling real-time monitoring and detection of suspicious activities.
Identifying Anomalies in Real-time
Big Data helps banks detect fraudulent activities by analyzing large volumes of transaction data in real-time. Machine learning algorithms are particularly effective in this area, as they can:
- Spot Unusual Patterns: These algorithms can identify transactions that deviate from a customer’s typical behavior.
- Flag Suspicious Activities: By recognizing these anomalies, banks can take immediate action to prevent fraud.
For instance, a sudden large transaction from an unfamiliar location might trigger an alert, prompting the bank to investigate further.
Reducing Fraud-related Losses
The financial benefits of using Big Data for fraud prevention are significant. By detecting and preventing fraud in real-time, banks can reduce losses and protect their reputation. Moreover, effective fraud prevention builds customer trust, as clients feel safer knowing their bank is proactively safeguarding their assets.
Regulatory Compliance and Risk Management
Regulatory compliance is a major concern for banks, and Big Data analytics is proving to be an invaluable tool in this area. By providing insights into potential compliance risks, Big Data helps banks avoid costly penalties and maintain their reputation.
Ensuring Regulatory Compliance with Big Data
Big Data analytics enables banks to meet regulatory requirements more effectively by:
- Monitoring Compliance in Real-time: Banks can track their compliance with regulations continuously, ensuring they are always in line with legal standards.
- Using Predictive Analytics: By predicting potential compliance issues, banks can address them before they escalate, reducing the risk of fines and penalties.
Stress Testing and Scenario Analysis
Stress testing and scenario analysis are critical components of risk management. Big Data allows banks to conduct these tests more thoroughly by simulating various economic scenarios and assessing their impact on the bank’s financial stability. This helps banks prepare for potential downturns and ensures they have the necessary safeguards in place.
Operational Efficiency and Cost Reduction
Big Data is not only enhancing risk assessment but also improving operational efficiency in banks.
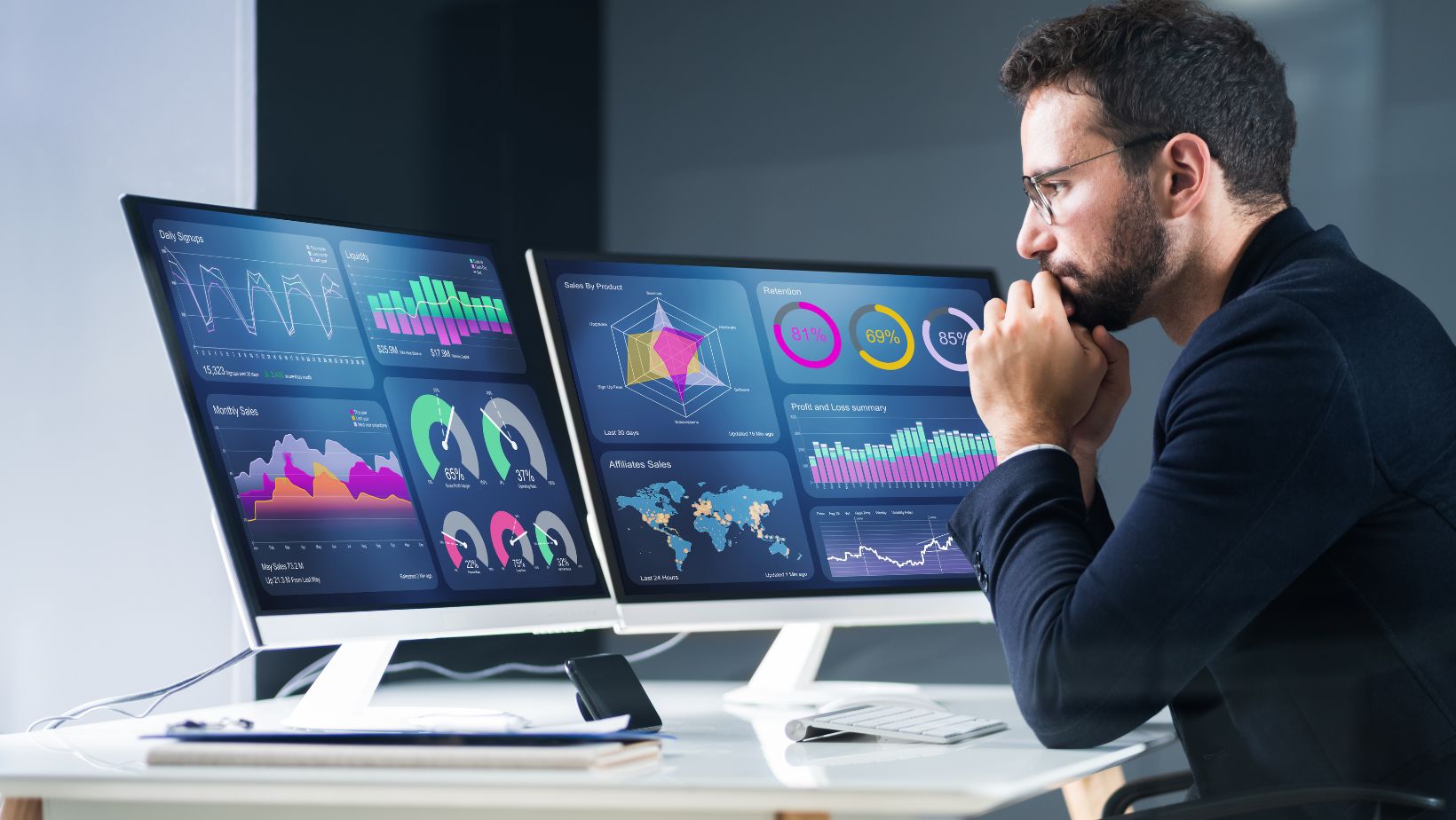
By automating routine tasks and optimizing resource allocation, banks can reduce costs and operate more effectively.
Automating Risk Assessment Processes
Big Data enables the automation of many risk assessment processes, which leads to:
- Reduced Human Error: Automated systems are less prone to mistakes compared to manual processes.
- Increased Efficiency: Automation speeds up risk assessment, allowing banks to respond to risks more quickly.
Optimizing Resource Allocation
With Big Data, banks can allocate resources more effectively by:
- Identifying Inefficiencies: Data analytics helps banks pinpoint areas where resources are being wasted.
- Implementing Cost-saving Measures: Banks can use these insights to streamline operations and reduce expenses.
Challenges in Implementing Big Data for Risk Assessment
While Big Data offers numerous benefits, its implementation comes with challenges that banks must address to maximize its potential.
Data Quality and Integrity
The accuracy of risk assessments depends heavily on the quality of the data being analyzed. Banks face challenges in ensuring that their data is clean, accurate, and up-to-date, as poor data quality can lead to incorrect risk assessments and flawed decision-making.
High Costs and Technical Requirements
Implementing Big Data solutions requires significant investment in technology and skilled personnel. Banks must weigh the costs of these investments against the potential benefits, ensuring they have the necessary resources and expertise to manage and analyze Big Data effectively.
The Impact of Big Data on Banking
Big Data is undeniably transforming the banking industry, particularly in the area of risk assessment. Providing more accurate, real-time insights enables banks to manage risks more effectively and operate more efficiently. As the banking sector continues to evolve, the role of Big Data will only become more prominent, driving further innovations and improvements in risk management practices.
Bryan Ziegenfuse, a recognized expert in financial planning and modeling, has noted the critical role of Big Data in enhancing the strategic capabilities of banks, highlighting its importance in the ongoing transformation of the industry. Looking ahead, banks that successfully integrate Big Data into their operations will be well-positioned to navigate the complexities of the modern financial landscape.